In industries such as electronics, aerospace, automotive, and defense, products routinely face complex dynamic loads during transportation and operation. Traditional vibration tables have long been the workhorses of environmental testing, simulating these conditions with established control systems. However, with the rapid advancement of artificial intelligence (AI), a pressing question arises: can AI technology truly be applied to vibration tables to transform their performance and functionality?
High-frequency vibration tables are critical in replicating real-world dynamic stresses. They need to precisely control parameters such as amplitude, displacement, and acceleration over a broad frequency range. Conventional control methods—primarily based on PID algorithms and fixed test profiles—work well under standard conditions. Yet, when faced with unpredictable load variations and non-linear responses, these traditional systems may struggle to maintain accuracy. This is where AI has the potential to make a significant impact.
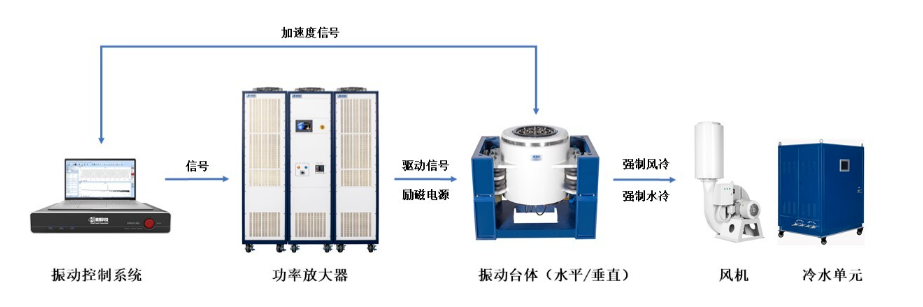
AI in Intelligent Control and Adaptive Modulation
One key area where AI could be beneficial is in intelligent control and adaptive modulation. By analyzing vast amounts of historical test data, machine learning algorithms could predict optimal control settings in real time, automatically adjusting the excitation parameters to maintain constant acceleration even when load conditions change. This approach not only enhances precision but also minimizes the need for manual intervention, thereby increasing efficiency and repeatability.
Real-Time Data Acquisition and Analysis
Another promising application is in data acquisition and real-time analysis. High-frequency vibration testing generates enormous volumes of data every second, and traditional methods can sometimes fall short in processing this information quickly. AI-powered data analytics, including deep learning models, can sift through these datasets, detect anomalies, and identify patterns that might indicate early signs of equipment degradation or failure. Such predictive capabilities could lead to proactive maintenance strategies, reducing downtime and extending the life of the testing equipment.
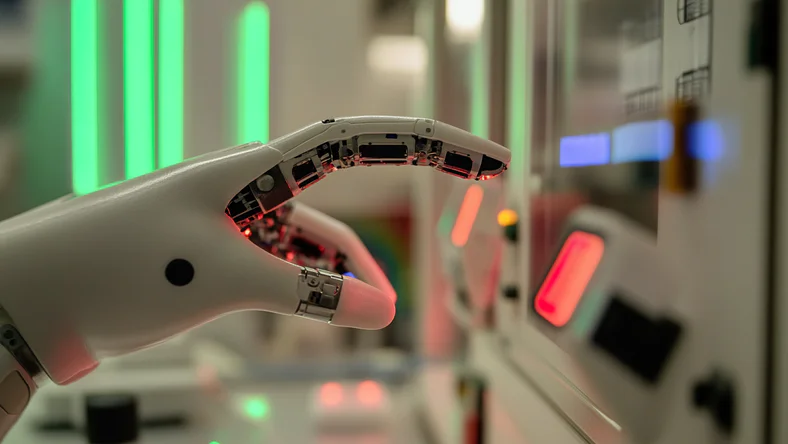
Multi-Axis Coordination and Synchronization
Beyond control and data analysis, AI could also facilitate multi-axis coordination. Modern vibration testing often requires simultaneous control of multiple axes to mimic real-world conditions accurately. An AI-based system could integrate sensor data from the X, Y, and Z axes, synchronizing them seamlessly to provide a more realistic and comprehensive dynamic load simulation.
Current Developments and Future Outlook
While several vibration testing products on the market have begun integrating smart control features and real-time feedback systems, full-scale adoption of AI remains in its infancy. Some current products offer a blend of traditional and intelligent features, such as adaptive PID controllers and automated centering mechanisms, which are the precursors to a fully AI-driven solution.
Looking forward, the potential of AI in vibration testing is immense. We may soon see the emergence of fully autonomous vibration tables that continuously learn and adapt to their testing environments, offering unprecedented accuracy and reliability. This evolution could transform environmental testing into a more predictive, efficient, and data-driven process, aligning with the broader trends of Industry 4.0.
Can AI Technology Be Applied to Vibration Tables? A Comprehensive Discussion
In industries such as electronics, aerospace, automotive, and defense, products routinely face complex dynamic loads during transportation and operation. Traditional vibration tables have long been the workhorses of environmental testing, simulating these conditions with established control systems. However, with the rapid advancement of artificial intelligence (AI), a pressing question arises: can AI technology truly be applied to vibration tables to transform their performance and functionality?
High-frequency vibration tables are critical in replicating real-world dynamic stresses. They need to precisely control parameters such as amplitude, displacement, and acceleration over a broad frequency range. Conventional control methods—primarily based on PID algorithms and fixed test profiles—work well under standard conditions. Yet, when faced with unpredictable load variations and non-linear responses, these traditional systems may struggle to maintain accuracy. This is where AI has the potential to make a significant impact.
AI in Intelligent Control and Adaptive Modulation
One key area where AI could be beneficial is in intelligent control and adaptive modulation. By analyzing vast amounts of historical test data, machine learning algorithms could predict optimal control settings in real time, automatically adjusting the excitation parameters to maintain constant acceleration even when load conditions change. This approach not only enhances precision but also minimizes the need for manual intervention, thereby increasing efficiency and repeatability.
Real-Time Data Acquisition and Analysis
Another promising application is in data acquisition and real-time analysis. High-frequency vibration testing generates enormous volumes of data every second, and traditional methods can sometimes fall short in processing this information quickly. AI-powered data analytics, including deep learning models, can sift through these datasets, detect anomalies, and identify patterns that might indicate early signs of equipment degradation or failure. Such predictive capabilities could lead to proactive maintenance strategies, reducing downtime and extending the life of the testing equipment.
Multi-Axis Coordination and Synchronization
Beyond control and data analysis, AI could also facilitate multi-axis coordination. Modern vibration testing often requires simultaneous control of multiple axes to mimic real-world conditions accurately. An AI-based system could integrate sensor data from the X, Y, and Z axes, synchronizing them seamlessly to provide a more realistic and comprehensive dynamic load simulation.
Current Developments and Future Outlook
While several vibration testing products on the market have begun integrating smart control features and real-time feedback systems, full-scale adoption of AI remains in its infancy. Some current products offer a blend of traditional and intelligent features, such as adaptive PID controllers and automated centering mechanisms, which are the precursors to a fully AI-driven solution.
Looking forward, the potential of AI in vibration testing is immense. We may soon see the emergence of fully autonomous vibration tables that continuously learn and adapt to their testing environments, offering unprecedented accuracy and reliability. This evolution could transform environmental testing into a more predictive, efficient, and data-driven process, aligning with the broader trends of Industry 4.0.
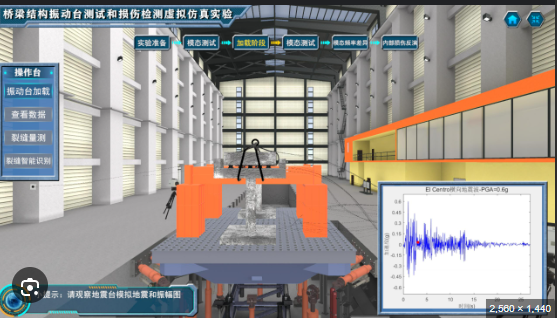
What Comes Next?
As AI continues to evolve, we must ask: How much autonomy should we allow AI in high-precision testing? Can AI-driven vibration tables maintain the same level of accuracy and consistency as traditional systems, or will human intervention still be necessary? Moreover, what challenges might arise in terms of data security, reliability, and industry regulations when AI takes over more control?
While AI offers exciting possibilities, its role in vibration testing is still an open discussion. Should we fully trust AI with critical test environments, or is a hybrid model the ideal solution? As the industry progresses, these questions will become even more relevant, shaping the future of vibration testing as we know it.